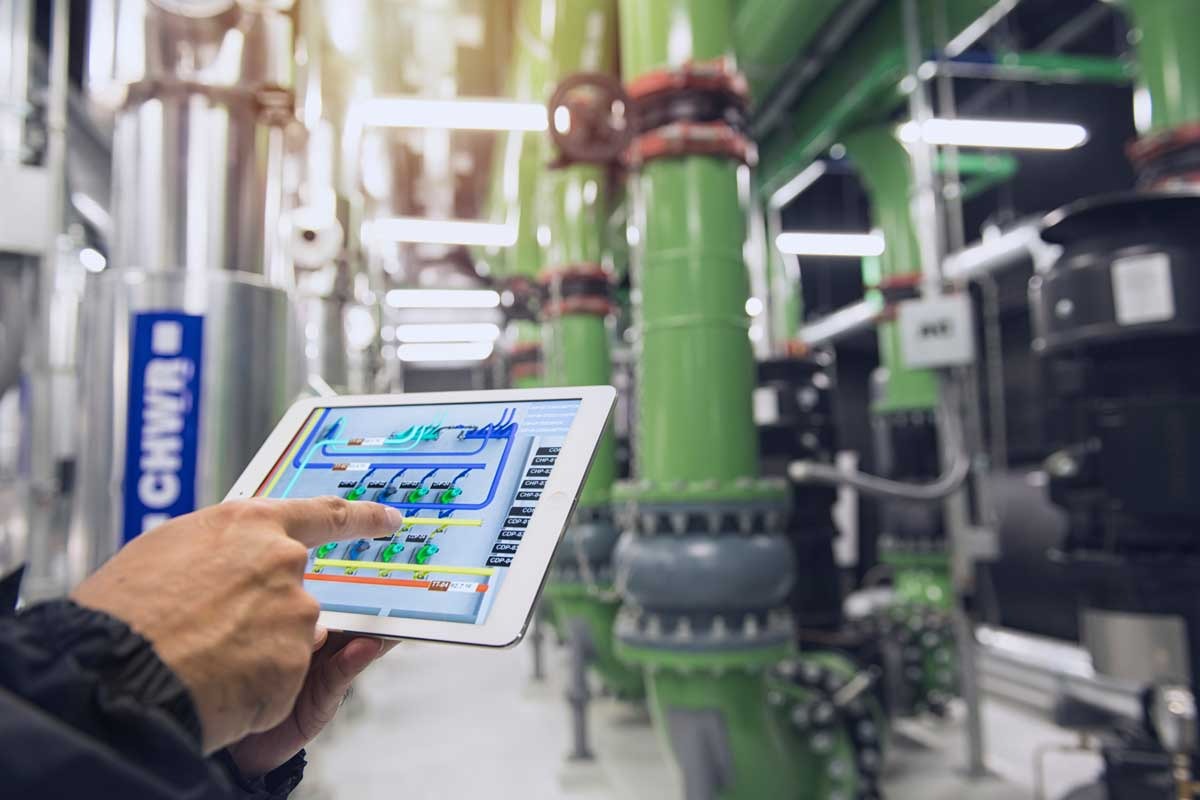
The present model has weaknesses. It might battle with correctly simulating the physics of a fancy scene, and should not have an understanding of specific occasions of induce and outcome. For example, anyone might take a Chunk outside of a cookie, but afterward, the cookie may well not Have got a Chunk mark.
a lot more Prompt: A white and orange tabby cat is noticed happily darting via a dense backyard garden, as though chasing some thing. Its eyes are wide and joyful since it jogs forward, scanning the branches, bouquets, and leaves since it walks. The path is narrow because it would make its way involving every one of the plants.
Curiosity-driven Exploration in Deep Reinforcement Studying through Bayesian Neural Networks (code). Successful exploration in significant-dimensional and steady Areas is presently an unsolved obstacle in reinforcement Discovering. Without effective exploration methods our brokers thrash all-around until finally they randomly stumble into fulfilling conditions. This is certainly enough in many easy toy tasks but inadequate if we want to apply these algorithms to complicated configurations with superior-dimensional motion spaces, as is widespread in robotics.
Most generative models have this basic setup, but differ in the details. Allow me to share 3 well known examples of generative model methods to give you a way of your variation:
Smart Choice-Building: Using an AI model is comparable to a crystal ball for viewing your foreseeable future. Using these types of tools assist in analyzing related info, spotting any pattern or forecast that can tutorial a company in building sensible conclusions. It entAIls fewer guesswork or speculation.
Ashish can be a techology specialist with 13+ a long time of practical experience and focuses primarily on Details Science, the Python ecosystem and Django, DevOps and automation. He focuses primarily on the look and shipping of essential, impactful packages.
This can be enjoyable—these neural networks are Discovering what the visual planet appears like! These models commonly have only about a hundred million parameters, so a network trained on ImageNet needs to (lossily) compress 200GB of pixel knowledge into 100MB of weights. This incentivizes it to find the most salient features of the data: for example, it'll probably understand that pixels close by are more likely to provide the similar coloration, or that the whole world is made up of horizontal or vertical edges, or blobs of different colors.
Prompt: This shut-up shot of a chameleon showcases its striking shade modifying capabilities. The history is blurred, drawing notice to your animal’s putting visual appearance.
GPT-3 grabbed the earth’s interest not merely because of what it could do, but on account of the way it did it. The striking soar in performance, Specially GPT-three’s ability to generalize across language jobs that it experienced not been particularly qualified on, didn't originate from much better algorithms (although it does depend closely on a sort IC design of neural network invented by Google in 2017, identified as a transformer), but from sheer size.
These parameters may be set as Portion of the configuration available through the CLI and Python bundle. Look into the Attribute Retail outlet Tutorial To find out more with regard to the available feature set turbines.
Examples: neuralSPOT contains several power-optimized and power-instrumented examples illustrating how you can use the above libraries and tools. Ambiq's ModelZoo and MLPerfTiny repos have even more optimized reference examples.
It could crank out convincing sentences, converse with humans, and perhaps autocomplete code. GPT-3 was also monstrous in scale—much larger than every other neural network ever created. It kicked off a whole new pattern in AI, one through which bigger is best.
Ambiq’s ultra-small-power wi-fi SoCs are accelerating edge inference in devices minimal by measurement and power. Our products allow IoT firms to provide answers which has a for much longer battery everyday living and much more elaborate, a lot quicker, and State-of-the-art ML algorithms right for the endpoint.
This contains definitions utilized by the rest of the documents. Of individual fascination are the following #defines:
Accelerating the Development of Optimized AI Features with Ambiq’s neuralSPOT
Ambiq’s neuralSPOT® is an open-source AI developer-focused SDK designed for our latest Apollo4 Plus system-on-chip (SoC) family. neuralSPOT provides an on-ramp to the rapid development of AI features for our customers’ AI applications and products. Included with neuralSPOT are Ambiq-optimized libraries, tools, and examples to help jumpstart AI-focused applications.
UNDERSTANDING NEURALSPOT VIA THE BASIC TENSORFLOW EXAMPLE
Often, the best way to ramp up on a new software library is through a comprehensive example – this is why neuralSPOt includes basic_tf_stub, an illustrative example that leverages many of neuralSPOT’s features.
In this article, we walk through the example block-by-block, using it as a guide to building AI features using neuralSPOT.
Ambiq's Vice President of Artificial Intelligence, Carlos Morales, went on CNBC Street Signs Asia to discuss the power consumption of AI and trends in endpoint devices.
Since 2010, Ambiq has been a leader in ultra-low power semiconductors that enable endpoint devices with more data-driven and AI-capable features while dropping the energy requirements up to Ambiq apollo4 10X lower. They do this with the patented Subthreshold Power Optimized Technology (SPOT ®) platform.
Computer inferencing is complex, and for endpoint AI to become practical, these devices have to drop from megawatts of power to microwatts. This is where Ambiq has the power to change industries such as healthcare, agriculture, and Industrial IoT.
Ambiq Designs Low-Power for Next Gen Endpoint Devices
Ambiq’s VP of Architecture and Product Planning, Dan Cermak, joins the ipXchange team at CES to discuss how manufacturers can improve their products with ultra-low power. As technology becomes more sophisticated, energy consumption continues to grow. Here Dan outlines how Ambiq stays ahead of the curve by planning for energy requirements 5 years in advance.
Ambiq’s VP of Architecture and Product Planning at Embedded World 2024
Ambiq specializes in ultra-low-power SoC's designed to make intelligent battery-powered endpoint solutions a reality. These days, just about every endpoint device incorporates AI features, including anomaly detection, speech-driven user interfaces, audio event detection and classification, and health monitoring.
Ambiq's ultra low power, high-performance platforms are ideal for implementing this class of AI features, and we at Ambiq are dedicated to making implementation as easy as possible by offering open-source developer-centric toolkits, software libraries, and reference models to accelerate AI feature development.
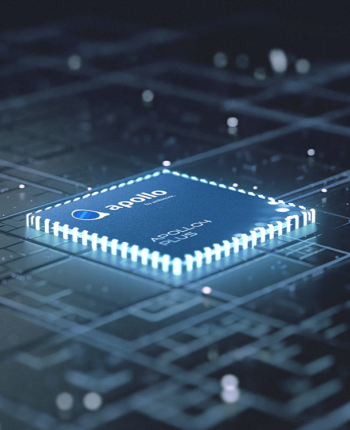
NEURALSPOT - BECAUSE AI IS HARD ENOUGH
neuralSPOT is an AI developer-focused SDK in the true sense of the word: it includes everything you need to get your AI model onto Ambiq’s platform. You’ll find libraries for talking to sensors, managing SoC peripherals, and controlling power and memory configurations, along with tools for easily debugging your model from your laptop or PC, and examples that tie it all together.
Facebook | Linkedin | Twitter | YouTube